This blog is an excerpt from the ebook: “A primer for data readiness for generative AI.” Download the complete version here.
[CTA_MODULE]
Generative AI sets itself apart from other forms of machine learning and artificial intelligence in its generative nature. Rather than merely analyzing data, generative AI produces new data in all forms of media – text, code, images, audio, video and more.
Under the hood, generative AI models are supported by machine learning models called artificial neural networks. Inspired by the architecture of brains, neural networks are designed to model complex, non-linear relationships using a graph data structure.
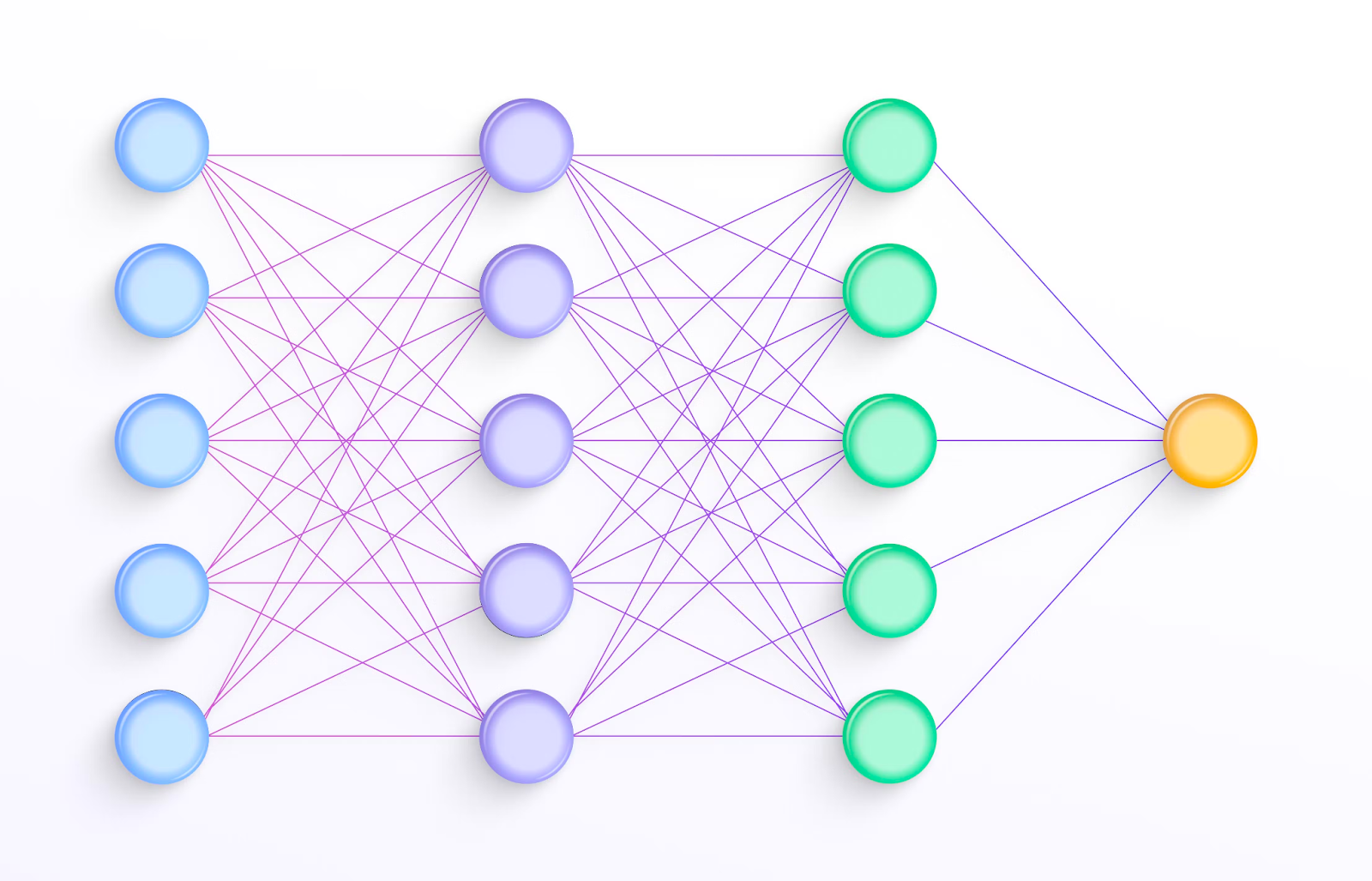
The key benefit of neural networks is that they learn relationships and patterns through exposure to examples instead of explicit programming. The output of a generative AI model is often refined through a process called reinforcement learning with human feedback (RLHF) – meaning that the model continuously refines itself on the basis of whether humans accept or reject its outputs.
A type of neural network model called a transformer forms the backbone of most modern generative AI models. Transformers can be massively parallelized and scaled, process inputs in a non-sequential manner (i.e. analyzing sentences, paragraphs and essays holistically instead of word-by-word) and support positional embeddings, an essential characteristic of modern large language models (LLMs).
Off-the-shelf transformer-based models trained on huge volumes of publicly available data are also known as base or foundation models. Most commercial applications of generative AI combine foundation models built by a third party with an organization’s proprietary data.
What are large language models?
Large language models (LLMs) are a type of generative AI that is trained on and produces text in response to prompts. This output typically takes the form of natural language, though LLMs can be used to generate text that follows other patterns, such as code, as well.
The “largeness” of an LLM comes from both the enormous volumes of data involved as well as the complexity of the model. Models such as ChatGPT are trained on petabytes of data collected from across the internet, consisting of trillions of tokens, or unique units of text (i.e. punctuation, characters, parts of words, words, phrases and more). These tokens are assigned unique identifiers and arranged into embeddings, or positions, in a massive, multidimensional space near positions of semantically and contextually similar tokens.
What generative AI is not: Despite its ability to mimic human language and other creative or intellectual output, a generative AI model fundamentally does not have any consciousness, emotions, subjectivity or semantic, human-like understanding of the data it ingests. Under the hood, like all machine learning, generative AI is just linear algebra, statistics and computation on a gargantuan scale.
Generative AI's distinctive nature marks a significant departure from other forms of artificial intelligence, expanding possibilities for creativity and problem-solving. Generative Ai paves the way for innovation across diverse domains, positioning itself as a key player in shaping the future of artificial intelligence and machine learning.
[CTA_MODULE]