In 2020, Deloitte declared that “the window for artificial intelligence (AI) competitive advantage was closing." Fast-forward to today and the message is even more urgent. In our recent global survey of senior IT and data science professionals, conducted by Vanson Bourne, 86 percent of respondents agreed that AI is the future, and organizations that do not utilize it will fail to survive.
Yet over half of organizations (55 percent) have only begun using machine learning (ML) and AI methodologies in the last six months. Moreover, only 14 percent of organizations consider their AI maturity "advanced." So what’s causing this disconnect?
While 85 percent of organizations are moving forward with AI models to help them strategically extract insights and take action, they are running into roadblocks — chiefly a lack of automation with their data and AI pipelines. Ninety percent of organizations indicated that their data processes are still manual, resulting in time-intensive processes requiring highly skilled resources that aren't available.
Organizations say that if they could automate just one process, the top choice would be data ingestion or data preprocessing/transformation, as shown in the chart below.
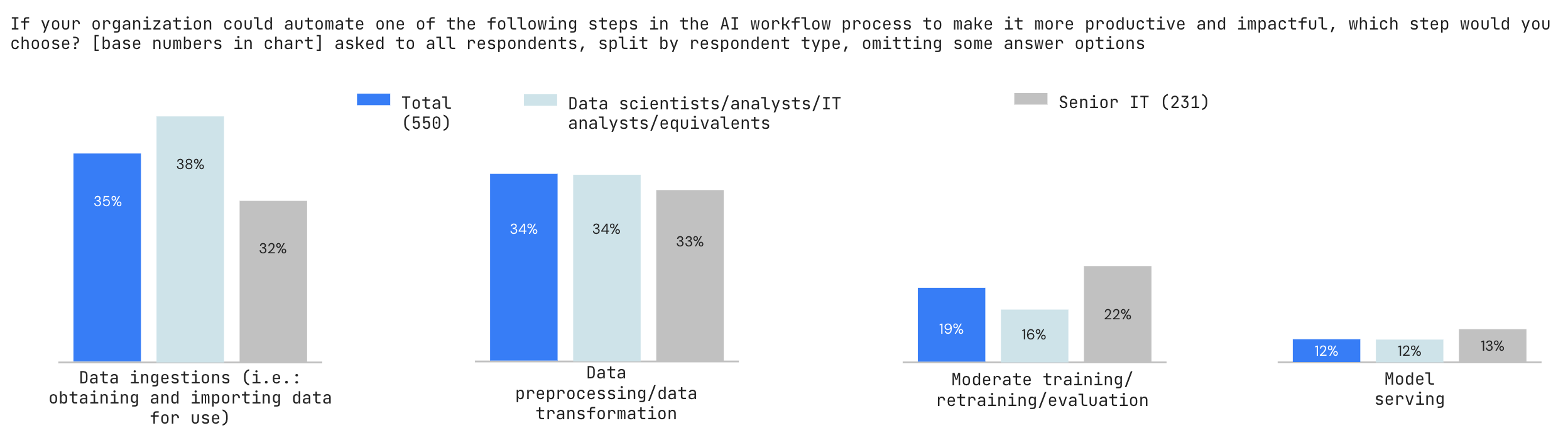
So companies understand the need for and value of AI. But to advance AI initiatives and maturity, organizations need to apply the following principles:
- Automate your data pipeline
- Free up your data scientists to focus on delivering high-value models and insights
- Educate stakeholders to increase trust in AI
Principle #1: Automate your data pipeline
Transforming data is complex. Technical data pipeline issues are where most companies get stuck or bogged down with AI initiatives. As a result, the majority of organizations say they face challenges. For example:
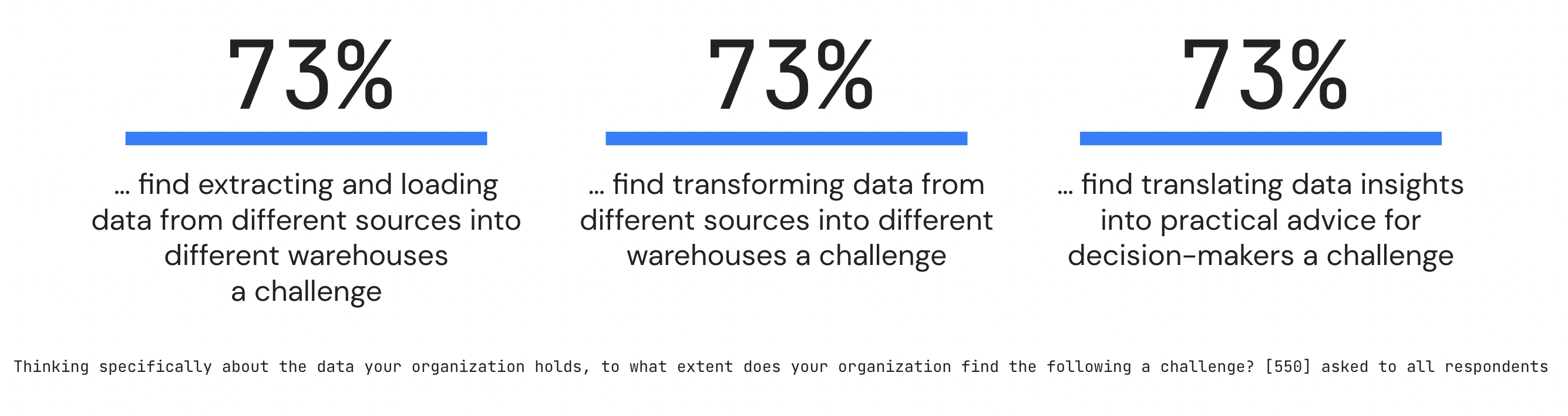
With data scientists currently spending an average of 70 percent of their time working with and preparing data versus building AI models, automating these types of data processes would alleviate many of the challenges organizations face. It would also free up data scientists to spend more time working with data rather than preparing it.
With greater automation, organizations can achieve greater scale and cost-efficiencies while saving time. More importantly, more automation allows data scientists to focus on solving complex problems that matter to the business rather than keeping data pipelines working.
Principle #2: Free up your data scientists to focus on high-value models and insights
The IT skills gap is a prominent challenge within organizations currently. Manual data and AI pipeline processes that require skilled data engineers and IT professionals put additional pressure on the scarce IT resources the company does have.
Organizations that aren't building AI models to increase analytical insights say that the two most common reasons center on a lack of skilled resources: They have the skills, but their attention is focused on other projects (46 percent), or they don't have the skills internally at all (43 percent).
Equally troubling is that some respondents indicated that they did not see the benefit of building AI models from business applications to make predictions and/or business decisions. If this is the perspective of an organization or senior IT professionals within the organization, it makes it much more challenging to get the necessary resources allocated to data and AI projects.
To move AI and ML projects forward and advance their maturity, organizations must first be educated on the advantages AI and data analytics can bring to the organization (increased competitiveness, better decision-making, greater productivity and so forth). Secondly, they need to find people with the right skills and let them focus on building machine learning models, not manual data pipeline processes. Eighty-seven percent of respondents agreed that data scientists and engineers were not being utilized to their full potential within their organization.
Principle #3: Educate stakeholders to increase trust in AI
Automation is essential given the volume of data necessary to build and run AI and ML models. Yet, the survey showed that technology is not being utilized to its full extent in decision making with people still heavily involved in this process. One of the biggest reasons people are still heavily involved is the concern around underperforming AI models that are built using inaccurate or low-quality data.
Poor data quality also degrades trust. In our survey, 86 percent of respondents agreed that their organization would struggle to fully trust AI to make all business decisions. This lack of trust means that AI may potentially never be used in its most true form and would also make it harder to achieve buy-in from stakeholders who hold the purse strings.
Poor data quality is also costly. Organizations say, on average, they are losing 5 percent of their global annual revenue due to underperforming AI programs that use low-quality data.
Stakeholders and business users must be made aware of the processes behind AI to fully understand how these decisions are made. But, it's also important that human involvement is focused on the right areas—such as improving data quality and the performance of AI models, which will lead to greater trust.
Automate your data pipelines with Fivetran
By automating your data pipelines, you can achieve:
- Time savings: Automated data pipelines adapt to schema changes and allow you to ingest multiple data sources into a centralized cloud-based data warehouse or data lake for data transformation in a completely automated fashion, which provides significant time savings.
- Cost-efficiency: With a consumption-based pricing model, you can actively manage your costs by replicating only the data that you need.
- Greater scale: Your skilled resources will be spending less time on manual, repetitive tasks, which means they can launch and manage more data initiatives.
- Better use of talent: Once the data pipelines are set up and scheduled, little interaction with Fivetran pipelines is required. This frees up data scientists and engineers to focus on building AI models and increasing their organization's AI maturity.
Having mature AI capabilities is increasingly important — and the fastest and best way to advance these capabilities is by automating your data ingestion and transformation processes.